The Ultimate Guide to Image Classification Labeling Tools
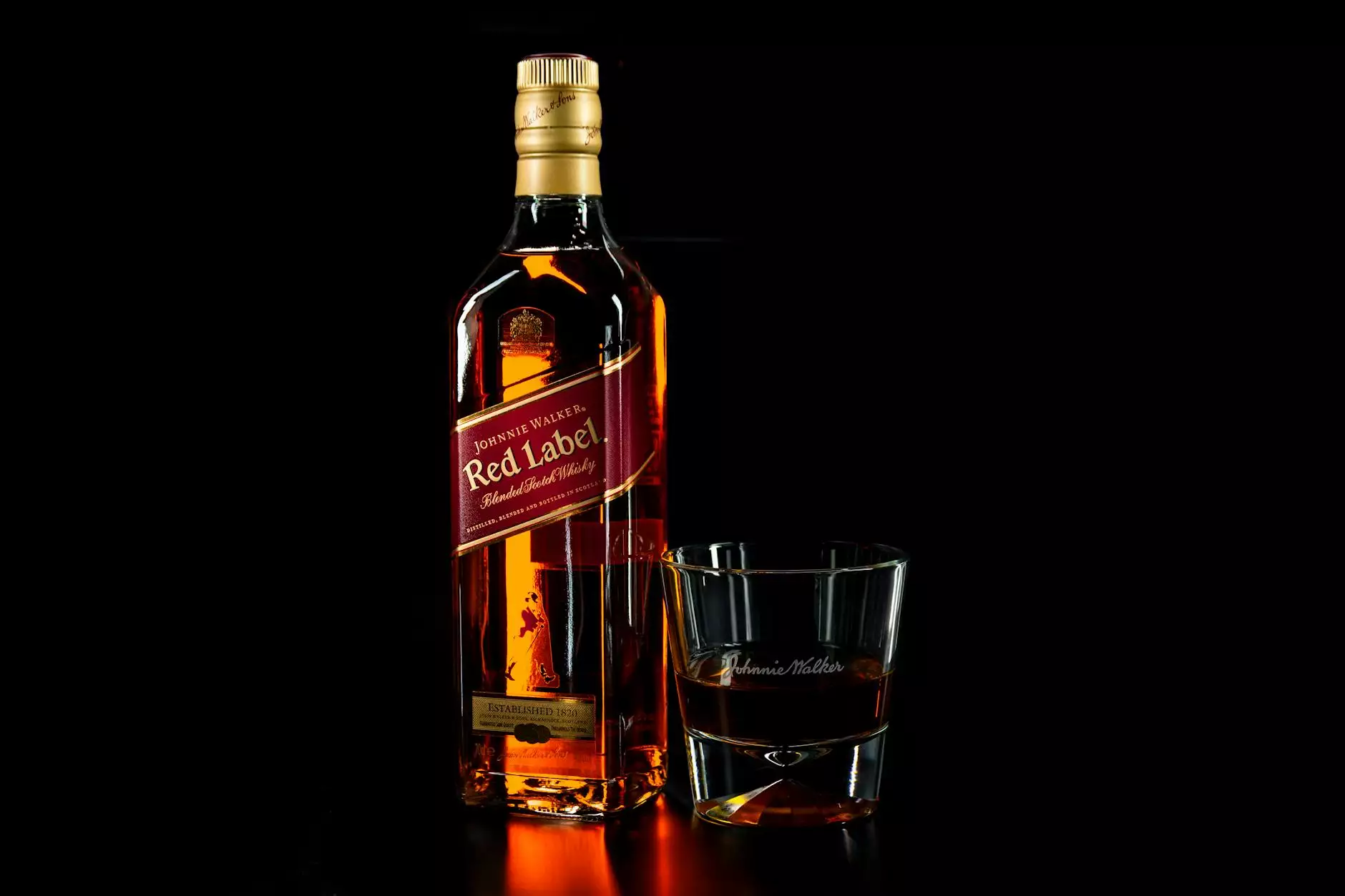
In today's digital landscape, data plays a pivotal role in driving decision-making and fueling innovations in various sectors. One of the critical components of effective data management is data annotation, which involves labeling data to provide context. Among the numerous tools available for this process, the image classification labeling tool stands out for its effectiveness in helping businesses categorize and analyze visual data. In this comprehensive guide, we will delve into the significance of these tools, their functionalities, and how they can transform your data annotation strategy.
What is an Image Classification Labeling Tool?
An image classification labeling tool is specialized software designed to assist users in the process of tagging and annotating images. This technology is integral for empowering machine learning models, particularly in applications related to computer vision. By enabling automatic identification and classification of images based on predefined categories, these tools streamline the data preparation phase, paving the way for more accurate insights and predictions.
Why Use Image Classification Labeling Tools?
Utilizing an image classification labeling tool can deliver numerous benefits to businesses across various domains:
- Efficiency: Automating the annotation process saves valuable time and resources, allowing teams to focus on high-level strategies.
- Accuracy: High-quality labeling minimizes errors, ensuring that models learn from correctly annotated data.
- Scalability: These tools can handle large volumes of images, making it easier to scale your data annotation needs as your project grows.
- Collaboration: Most platforms support collaborative features, enabling multiple users to contribute effectively to the annotation process.
- Customizability: Organizations can often customize labels and annotation workflows to align with specific project requirements.
Key Features of an Efficient Image Classification Labeling Tool
When selecting an image classification labeling tool, it's essential to consider several key features that enhance usability and effectiveness:
1. User-Friendly Interface
A straightforward and intuitive interface ensures that users can navigate the tool with ease, regardless of their technical expertise. A well-designed dashboard can significantly improve the user experience and reduce the learning curve.
2. Automated Image Labeling
The incorporation of machine learning algorithms for automated image labeling speeds up the annotation process. By pre-labeling images, these tools provide initial suggestions that can be manually adjusted, reducing the overall workload.
3. Compatibility with Various Data Formats
It’s crucial for an image classification labeling tool to support multiple image formats, including JPEG, PNG, and TIFF. This flexibility allows businesses to work with diverse data sets, ensuring comprehensive analysis.
4. Integration with Other Tools
Seamless integration capabilities with existing platforms such as project management, machine learning frameworks, and data management tools can enhance productivity by streamlining workflows.
5. Quality Control Mechanisms
Robust systems for quality assurance help maintain high labeling standards by implementing review stages where annotations can be vetted and corrected before being finalized.
Best Practices for Effective Image Classification
Adopting best practices in data annotation can lead to higher quality outputs from your image classification labeling tool. Here are some techniques to consider:
1. Define Clear Annotation Guidelines
Establishing clear, uniform guidelines for labeling is essential. This includes defining category descriptions and examples to ensure consistency across annotations. All team members should adhere to these guidelines to avoid ambiguity in labels.
2. Use Pre-Existing Datasets for Training
Leverage publicly available datasets to train your annotators and give them a benchmark to achieve. Familiarizing them with certain standards will improve their accuracy and understanding of the project requirements.
3. Implement Regular Feedback Loops
Creating communication channels for feedback on labeling accuracy can foster improvement. Regular team meetings to review common challenges and errors will encourage collaborative learning.
4. Monitor Annotation Quality
Use built-in tools or external systems to analyze how annotation performance evolves over time. This systematic review can enable early detection of declining quality, allowing for prompt corrective measures.
Impact of Image Classification on Business Outcomes
The implications of effective image classification reach far beyond simple categorization. Here’s how it influences various business outcomes:
A. Improved Decision-Making
By providing accurate data through image classification, businesses can make informed decisions based on solid evidence. Whether in healthcare, retail, or security, the ability to classify images allows organizations to identify trends, anomalies, and actionable insights promptly.
B. Enhanced Customer Experience
In sectors such as e-commerce, image classification aids in personalizing user experiences by accurately displaying products relevant to customers' preferences and previous interactions, ultimately boosting conversion rates.
C. Increased Operational Efficiency
With a faster and more efficient data annotating process, resources are better allocated to strategic initiatives rather than redundant tasks, ensuring optimal utilization of time and talent.
D. Competitive Advantage
Organizations that invest in a competent image classification labeling tool can develop superior AI models, thus gaining a competitive edge by improving performance in areas such as fraud detection, automated surveillance, or targeted marketing.
Integrating Image Classification with AI and Machine Learning
The surge of artificial intelligence (AI) and machine learning (ML) technologies has made image classification tools even more essential. Here's how they complement each other:
AI algorithms, particularly deep learning neural networks, rely heavily on well-annotated datasets to perform optimally. As the models are trained using these datasets, accurate labeling becomes crucial for them to recognize patterns and make predictions. Combining robust image classification labeling tools with AI/ML technologies allows organizations to:
- Enhance Model Accuracy: Properly classified images lead to better model training and, ultimately, more accurate predictions.
- Facilitate Continuous Learning: As new data becomes available, continuous annotation helps in improving model performance progressively.
- Drive Innovation: Using these technologies in conjunction fosters new use cases and solutions that can transform industries.
Conclusion
In conclusion, an image classification labeling tool is not merely a software requirement but a strategic asset for businesses aiming to leverage visual data effectively. By automating the annotation process, ensuring high-quality outputs, and integrating with advanced technologies, organizations can unlock significant efficiencies and insights that were previously beyond reach.
The landscape of data annotation is ever-evolving, and companies like KeyLabs.ai are at the forefront, providing cutting-edge data annotation tools and platforms that empower businesses to derive maximum value from their data. Embracing these technologies will prepare your organization for a future where data-driven decision-making is imperative for success.